Natural selection driven through interspecific and intraspecific competitive competition is a fundamental evolutionary mechanism which has led to the large diversity and complexity among species that inhabit Earth. Modern AI research has shown that this process can be replicated to a large extent in AI research. Machines have been able to reach superhuman performance through competitive multi-agent reinforcementlearning environments (RL).
However, designing multi-agent RL environments that foster the development of useful and interesting agent skills can be time-consuming and laborious. Domain randomization is a common approach to single-agent environments. This involves the agent being trained in a wide range of randomly generated environments. Recent work has improved this process by using automatic environment curricula techniques. These adapt the environment distribution during training to maximize number of environments that produce stronger and better skills.
The new paper AutoDIME: Automated Design of Multi-Agent EnvironmentsOpenAI researchers explore automatic environment design in multi-agent environments with RL-trained teachers that sample environments to maximize student learning. The research shows that intrinsic teacher reward is a promising approach to automating multi-agent environment design.
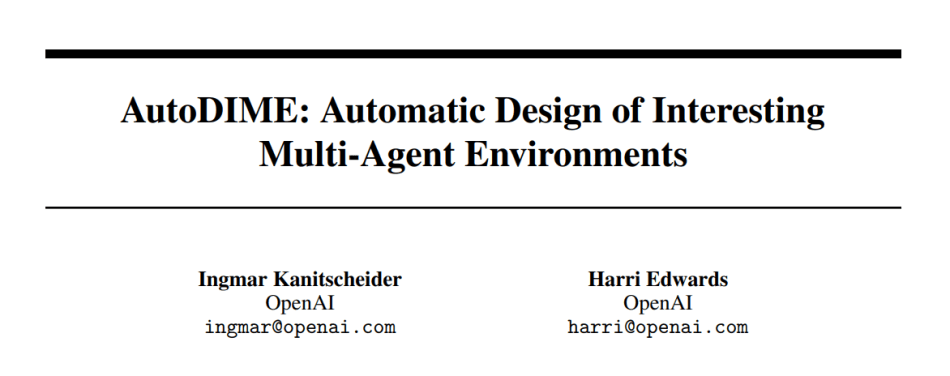
The team summarizes their main contributions by:
- We have shown that intrinsic rewards for teachers that compare student behavior to some prediction can lead faster skill emergence in multiagent Hide and Seek and quicker student learning in a single agent random maze environment.
- We develop an analog to the noisy TV problem for automatic environmental design and analyze the susceptibility intrinsic teacher rewards uncontrolled stochasticity in an agent random-maze environment. We find that value prediction error, and to a lesser extent policy disagreement, are susceptible to stochasticity. However, value disagreement (teacher rewards that measure disagreement among a set of student value function with different initializations) proves to be more resilient.
The teacher-student learning (TSCL), a training scheme for teacher-student curriculum, is used by researchers. It allows RL-trained teachers and students to see the environments where student agents are trained. Teachers are rewarded for creating learning environments that allow their students to excel. The teacher will first experience the environment in the beginning of a student episode. Then, the student policies and teacher reward are rolled out.
The team discovered two advantages to conditional sampling. They used it for their teacher sample strategy.
The team compared the effect of trained teachers on student learning with baseline training using a uniform or stationary environment distribution in their evaluations. The MuJoCo (Multi-Joint Dynamics with Contact) physics engine was used to simulate environments, including a modified Hide and Seek environment and a single-agent random maze environment.
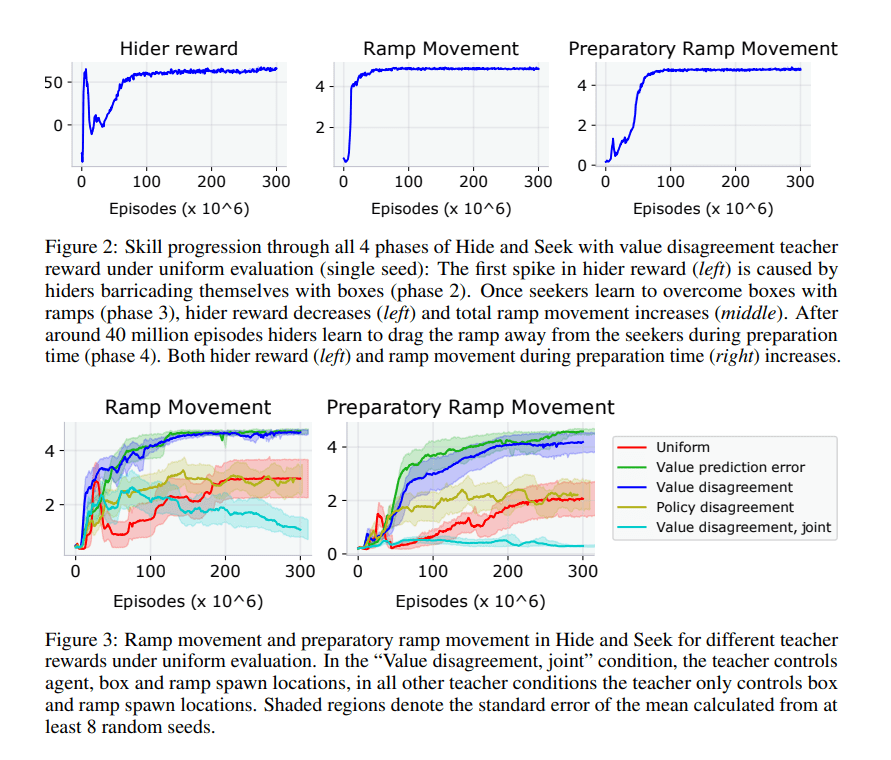
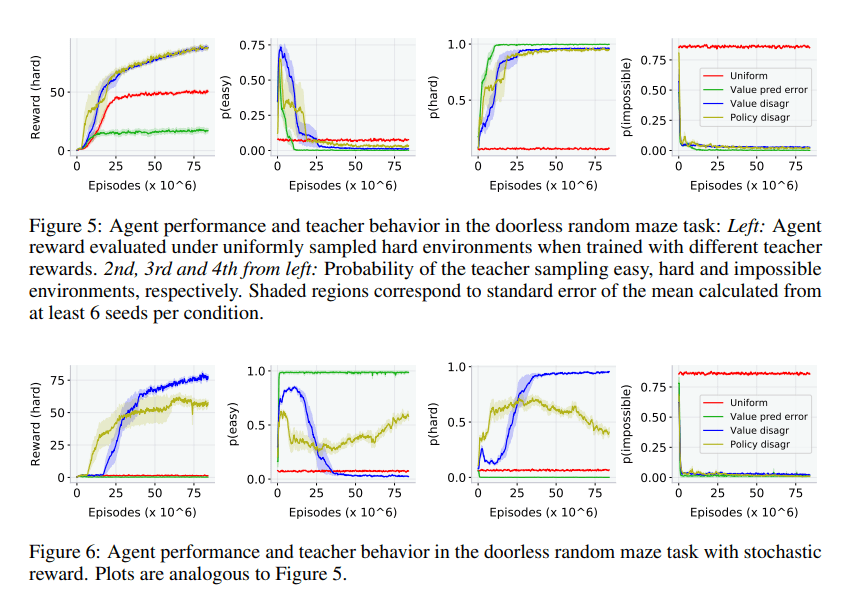
The team summarized their findings as follows:
- Value prediction error or value disagreement leads to faster and more reliable skill discoveries in multi-agent hide & seek than uniform sampling and policy disagreement.
- For both multi-agent environments as well as environments with stochasticity, value disagreement can be a promising teacher reward.
- Many teacher reward schemes that were previously proposed fall prey to adversarial situations, where the teacher reward can also be decoupled with genuine student learning progress.
- A teacher who is well-designed can help the student explore learnable environments.
The results show that intrinsic teacher rewards, and value disagreement in particular, are promising approaches to automating multi-agent environments.
The paper AutoDIME: Automated Design of Multi-Agent Environments It is on arXiv.
Author| Editor: Michael Sarazen
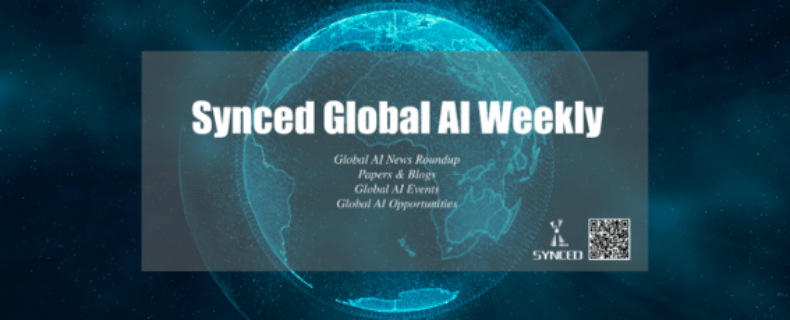
We know you don’t want any news or research breakthroughs to be missed. Subscribe to our popular newsletter Synced Weekly AI Weekly to get weekly AI updates.